New inspection method improves confidence in 3D laser powder bed printing
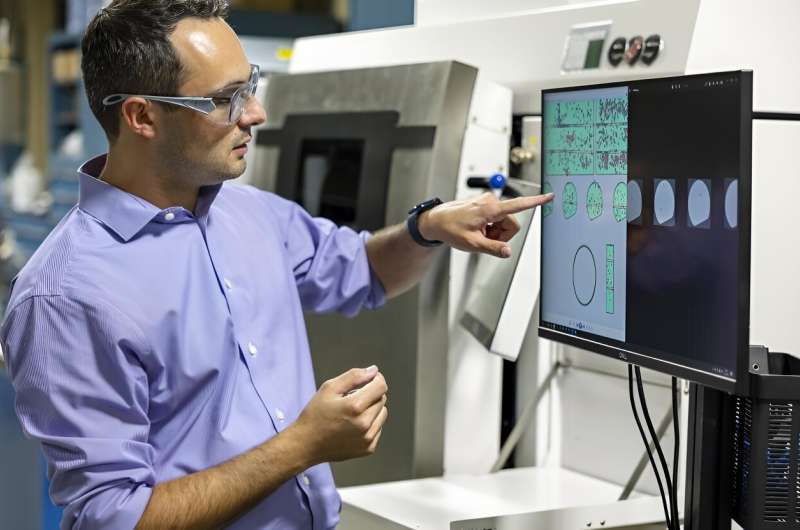
Researchers from the Department of Energy’s Oak Ridge National Laboratory improved flaw-detection to increase confidence in 3D-printed metal parts using laser powderbed fusion.
The energy, aerospace and nuclear industries can create complex parts from a wide range of materials using additive manufacturing. The technology is not widely used due to the difficulty of checking the product accurately and thoroughly. Conventional inspection methods might not detect flaws that are embedded deep within the layers of the printed part.
Researchers at ORNL developed a technique that combines the inspection of a printed part after its construction with data collected by sensors during the print process. This data is then used to train a machine learning algorithm that can identify defects in the product. This framework is even more important because it allows operators to be sure that they can detect flaws as accurately as with traditional methods, which require more time and effort.
“We can detect flaw sizes of about half a millimeter—about the thickness of a business card—90% of the time,” said ORNL researcher Luke Scime. “We are the first to assign a numerical value to the degree of confidence that can be achieved for flaw detection in situ (in the process).” This reflects the confidence that is shown in the safety and reliability of the product.
Laser powder bed-fusion is the most popular metal 3D printing process. It uses a high energy laser to melt metal powder which has been spread over a build plate. The build plate is then lowered before the system spreads out and melts a new layer, slowly building the designed product.
Engineers know that the material will have flaws.
Zackary Snow, an ORNL researcher, said: “For normal manufacturing we know where to look and how to locate them.” “(Operators know) the probability they can detect flaws a certain size so they know how many samples to inspect in order to get a sample representative.”
3D printing does not enjoy the same level of confidence.
Snow explained that without a part number, it is difficult to qualify or certify the parts. It’s one of additive manufacturing’s biggest obstacles.
Recently, ORNL researchers and RTX published a paper in Additive Manufacturing, explains their process to reach a 90% detection while reducing the likelihood of false positives that can lead to the scrapping or good products.
RTX, an aerospace and defense firm, designed a similar part to one that it produces to allow for realistic flaws to be seen. RTX then 3D printed the part several times, monitoring the build using a standard near infrared and a visible light camera. Both RTX researchers and ORNL researchers performed quality checks using X-ray computerized tomography (also known as CT scans).
With the assistance of RTX experts, ORNL additive manufacturers aligned the images into a layered pile, which essentially became a textbook for machine-learning algorithms. During training, an algorithm first labeled flaws by using CT scan images. A human operator then annotated all the remaining data based on visual clues from the data collected during printing. Human feedback is used to continue training the software so it recognizes faults more accurately every time. This novel approach was based on previous ORNL innovations in deep-learning frameworks and in-situ monitors. This will eventually reduce the need for humans to inspect manufacturing.
Snow explained, “This gives CT-level confidence in the absence of CT.” CT imaging and analysis is a common way to check some 3D printed parts. However, it can be expensive because of the extra time and expertise required. CT is also limited in its use because it can’t penetrate dense metals.
Scime explained that the algorithm can be trained to produce a quality analysis in a matter of days when it is applied to the same design, consistently produced with the exact same materials and methods. The software will also incorporate all the information it has learned from different constructions and designs, allowing it to check unfamiliar products.
ORNL’s inspection framework may help expand the use of additive manufacturing. Snow says that additive manufacturing, which involves the use of statistically validated quality control, could make it possible to mass-produce products such as car parts.
The types of parts which can be printed in 3D could also expand. It allows for more design freedom when you know how small the flaws are. This is important because the industry is already headed toward larger print volumes and faster print rates—meaning more lasers interacting to create bigger parts with different types of flaws, said Brian Fisher, senior principal engineer for additive manufacturing at RTX’s Raytheon Technologies Research Center.
“You can really start to save time and money and make a business case when printing larger assemblies—except those are also the hardest to inspect today,” Fisher said. “We have a vision that with additive manufacturing, we can produce large, complex parts made of dense materials which are traditionally very expensive and difficult to inspect.
The ORNL team then trains the deep learning algorithm to distinguish between different types and causes of flaws.
Further information
Zackary Snow and colleagues, Scalable non-destructive in situ evaluation of additively produced components using sensor fusion and machine learning. Additive Manufacturing (2023). DOI: 10.1016/j.addma.2023.103817
Oak Ridge National Laboratory
Citation:
New inspection method boosts confidence in laser powder-bed fusion 3D printers (2023, October 26).
Retrieved 26 October 2023
from https://techxplore.com/news/2023-10-method-confidence-laser-powder-bed.html
This document is protected by copyright. No fair trade is allowed, except for private research or study.
No part of the website may be reproduced or copied without written permission. This content is only provided as information.